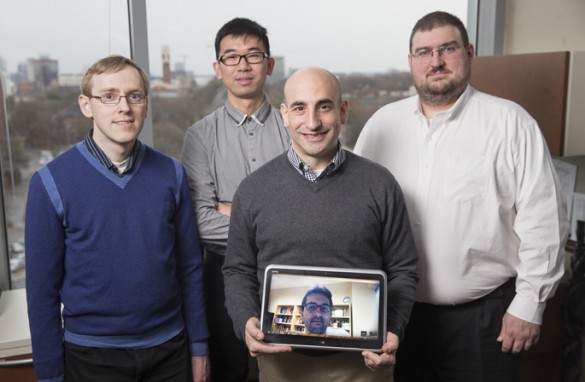
A new study from Vanderbilt introduces an adroit and flexible strategy to quash attacks on patient privacy and aid the flow of vital research data.
The study, which appeared recently in PLOS ONE, treats publishers and recipients of de-identified patient data as potential competitors whose prospective benefits, gains and losses can be estimated in dollars.
Patient records represent rich fuel for the advancement of science and medicine, and research funding agencies, along with drug and medical device companies, are ever more keen to see these data published and available for study — within the bounds of patient privacy. Obliging hospitals, health care payers and electronic medical record companies churn out patient records in de-identified form, and some make good money doing it.
Surreptitiously re-identified records could be used to discriminate against or otherwise prey upon patients. The most likely type of attacker would attempt to link public records — voter registration records, for example — to de-identified records, seeking as many unique (or close to unique) matches as possible.
Using mathematical models in the form of game theory, and a laptop computer, doctoral candidate Zhiyu Wan, Bradley Malin, Ph.D., Yevgeney Vorobeychik, Ph.D., and co-authors show it’s possible to smash a would-be privacy attacker’s expectations to zero while sharing much more research data than is typically shared today.
What’s more, the paper’s embedded case study of genomic data sharing suggests that currently favored patient de-identification practices are likely to leave privacy attack risks on the table. The authors’ re-identification game, as it’s called, can be wielded by publishers to remove this risk.
Consideration of real-world incentives has apparently been largely absent from theoretical work on patient de-identification. To the authors’ knowledge, this is the first study to apply game theory to potential data-oriented attacks on patient privacy.
“Some people will find this off-putting. It may be difficult to convince a regulator that balancing the need to protect privacy with sharing data for research purposes should be treated as if it’s a game,” said Malin, associate professor of Biomedical Informatics and Computer Science and director of the Health Information Privacy Laboratory.
The problem that haunts de-identification is that to strip out or partially obscure so-called quasi-identifiers — age, race, zip codes, etc. — is to significantly degrade a record’s scientific and public health utility.
There’s a simple recipe publishers can follow that indiscriminately suppresses all sorts of quasi-identifiers, and because it has the virtue of conferring safe harbor from federal penalties, it’s popular. Publishers are free to devise more discriminating strategies, but they understandably tend to prefer safe harbor.
The re-identification game lets publishers at once optimize the value of a de-identified data set and forestall attacks from rational adversaries. The game can accommodate different incentives leading to vastly different payoff levels. To demonstrate the game, the authors use grant dollars and fines to measure benefits, gains and losses.
In the no-risk version of the game, the publisher zeroes-out the attacker’s incentive as data are released in the game’s opening move.
As Vorobeychik notes, this is a somewhat artificial variant of the basic game, which has the publisher instead putting slight amounts of risk in play in order to optimize the value of the published data.
“But with the no-risk version there’s no downside and you can still share more data” than safe harbor, said Vorobeychik, assistant professor of Computer Science and Computer Engineering.
As currently set out, the game applies to structured data only — that is, numerical or categorical data typically arrayed in rows and columns.
The policy discussion would appear to be stuck in limbo. Enterprising journalists and academics, Malin among them, have found vulnerabilities in patient de-identification methods. But there have been no reports of anyone ever attacking these data with intention to harm.
Could the re-identification game enliven the policy discussion, help bring it down to earth?
“If we can do something to influence the way people think about data sharing and privacy, I think that would be a good contribution to the work,” Malin said.
Other authors include, from Vanderbilt, Weiyi Xia, M.S., Ellen Wright Clayton, M.D., J.D., and Raymond Heatherly, Ph.D., and, from the University of Texas at Dallas, Murat Kantarcioglu, Ph.D., and Ranjit Ganta Ph.D.
The research was supported by National Institutes of Health grants HG006844, HG006385 and LM009989, and by the National Science Foundation.