A research team at Vanderbilt University Medical Center (VUMC) will develop a crowdsourcing solution for generating a wide range of labeled data sets from electronic health records (EHRs).
This pilot project will solicit contributions from VUMC medical students and clinical personnel (nurses, residents and fellows), paying them to label EHR data.
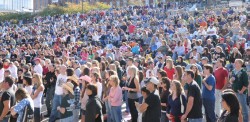
The work will be assisted by a $316,000 grant (CA203708-01) from the National Institutes of Health (NIH), under the research agency’s Big Data to Knowledge initiative.
EHRs collectively contain troves of data useful for statistically modeling health care and supporting the development of clinical prediction systems. Supervised learning is a machine learning technique using labeled data sets to generate predictive models automatically. Some EHR data can be automatically extracted and labeled for supervised learning. But other data extraction and labeling tasks require expertise and judgment, and that’s where crowdsourcing could help.
“If you want to label things like why a patient was readmitted to the hospital or why a hospital discharge was delayed, these more complex labels can’t be automatically inferred without introducing significant error rates. But manual chart review is time consuming, making it hard to create enough labels. If we’re going to continue to develop better clinical decision support, we need more labels,” said the project’s principal investigator, Daniel Fabbri, Ph.D., assistant professor of Biomedical Informatics and Computer Science.
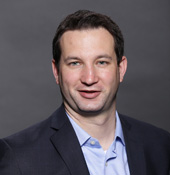
According to Fabbri, the cost for manual chart review at Vanderbilt is more than $100 per hour per worker.
“Through crowdsourcing, our goal is to make the task of producing these labels more cost efficient and more scalable, so that we can produce more labels and use them to build more efficient, accurate and robust clinical machine learning prediction models,” Fabbri said.
To safeguard patient privacy, all clinical records reviewed by labelers will be de-identified, and VUMC students and clinical staff will sign data use agreements to qualify as labelers. A VUMC team has already developed crowdsourcing software and an EHR search engine for the project.
Fabbri’s team is now looking for supervised learning projects to support. If you’re a Vanderbilt researcher doing IRB-approved studies and you’re seeking manually labeled clinical data, consider contacting Fabbri at Daniel.Fabbri@Vanderbilt.edu.
In a later phase of the project, the team will issue a general invitation to VUMC medical students and clinical staff to enroll as labelers.
Fabbri’s co-investigators include Joshua Denny, M.D., MS, Thomas Lasko, M.D., Ph.D., Bradley Malin, Ph.D., Laurie Novak, Ph.D., MHSA, and Yevgeniy Vorobeychik, Ph.D., MSE.