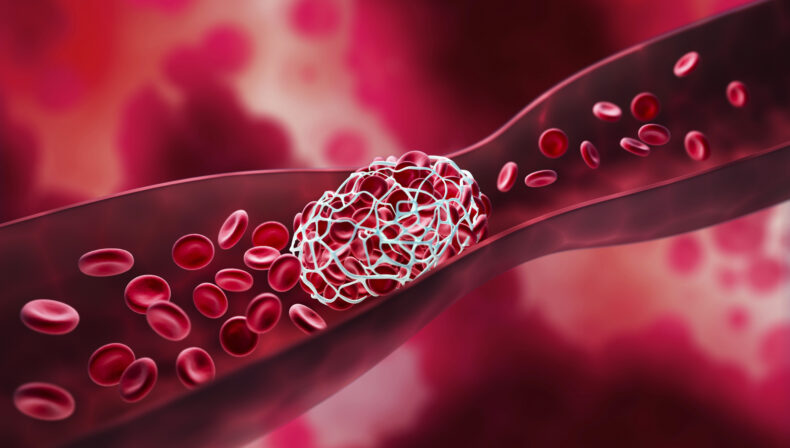
Considered a leading cause of preventable in-hospital deaths, hospital-acquired venous thromboembolism (HA-VTE) is deep vein thrombosis and pulmonary embolism that occurs during a hospital stay as a complication of acute medical or surgical admission. Benjamin Tillman, MD, Benjamin French, PhD, and colleagues, seeking improved prevention, have developed an automated algorithm to predict patients’ risk of HA-VTE.
As reported in Research and Practice in Thrombosis and Haemostasis, their project used machine learning regression analysis on three years of data from Vanderbilt University Medical Center (inpatients ages 16 years and older). In testing on the ensuing two years of VUMC data, the team shows that the algorithm exhibits excellent prediction performance across VUMC’s diverse inpatient population, achieving a C statistic of 0.897, indicating very high accuracy in distinguishing patients who turned out to have experienced HA-VTE from those who did not. Involving 25 readily available patient characteristics from the electronic health record, the team’s risk model is distinguished from several other HA-VTE risk models by the ability to provide real-time risk assessments that run in the background, without the need for manual input from health care providers.
For further evaluation of a model such as this, next steps would include external validation (again using observational data) followed by a prospective pragmatic trial to measure any patient outcome benefits.
Others on the study include Henry Domenico, MS, Ryan Moore, MS, Daniel Byrne, MS, Colleen Morton, MBBCH, and Amanda Mixon, MD, MSPH. The project was supported by the National Institutes of health (grants R01HL164482, UL1TR002243).