Researchers at Vanderbilt University Medical Center and the University of Calgary have developed a novel computational genetics approach to enhance the discovery of disease risk genes. Building on their previous work, the investigators have now integrated distant genetic variants into transcriptome-wide association studies (TWAS).
The new method, called transTF-TWAS, outperforms other existing TWAS approaches for identifying disease-associated genes, the investigators reported Nov. 13 in the journal Nucleic Acids Research.
Xingyi Guo, PhD, associate professor of Medicine in the Division of Epidemiology at VUMC, led development of the approach with Quan Long, PhD, associate professor of Biochemistry and Molecular Biology at the University of Calgary.
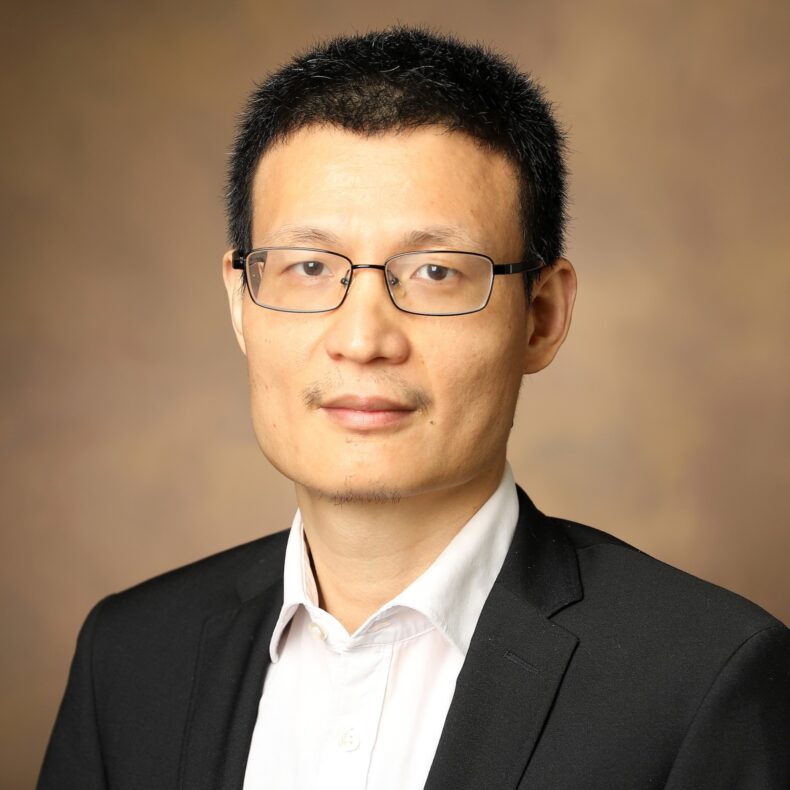
Genome-wide association studies (GWAS) have identified thousands of genetic variants associated with a range of complex diseases, including cancers. About 90% of the risk variants, however, are located in “noncoding” regions of the genome — they are not located in genes that code for proteins, suggesting they may affect disease risk by dysregulating gene expression.
TWAS analyses combine disease GWAS and gene expression data, such as from the Genotype-Tissue Expression project, aiming to identify disease risk genes.
Guo and colleagues previously developed sTF-TWAS, an approach that integrated transcription factors (TF), proteins involved in regulating gene expression, into the analysis and outperformed other TWAS methods for identifying cancer and other disease susceptibility genes. That method focused on “cis-variants” — variants located very close to the gene they regulate.
Now, the investigators have integrated TF-linked “trans-variants” — variants located far away from the gene they regulate — into the transTF-TWAS method.
“In TWAS, incorporating trans-variants into gene expression prediction model building is challenging due to the statistical burden of their overwhelming numbers,” Guo said. “Leveraging mechanistically based TF-linked trans-variants offers a novel strategy to improve gene expression predictions and enhance the discovery of disease risk genes.”
The researchers applied this new approach to large GWAS datasets for breast, prostate and lung cancers, and three brain disorders.
Their analysis revealed 887 putative cancer susceptibility genes, including 465 in regions not reported by previous GWAS, with many known to have roles in cancer development or cell proliferation. The findings shed new light on several key TF regulators and their associated regulatory networks.
The transTF-TWAS method is a valuable addition to tools for discovering disease risk genes, the researchers note.
Other VUMC study authors are Wanqing Wen, MD, MPH, Jie Ping, PhD, Qing Li, PhD, Linshuoshuo Lyu, Zhishan Chen, PhD, Jirong Long, PhD, Qiuyin Cai, MD, PhD, Xiao-Ou Shu, MD, PhD, MPH, and Wei Zheng, MD, PhD, MPH. The research was supported by the National Institutes of Health (grants R37CA227130, R01CA269589), New Frontiers in Research Fund, China Scholarship Council, Alberta Innovates, Eyes High, and Canada Foundation for Innovation.