Algorithm boosts protection of study subjects’ anonymity
A new study by Vanderbilt researchers sets out a quick solution to better protect the anonymity of research subjects participating in genome-wide association studies (GWAS).
The authors are equally at pains to protect the scientific utility of anonymized data, so as not to discourage researchers from borrowing GWAS data to validate a prior study or to open new lines of inquiry.
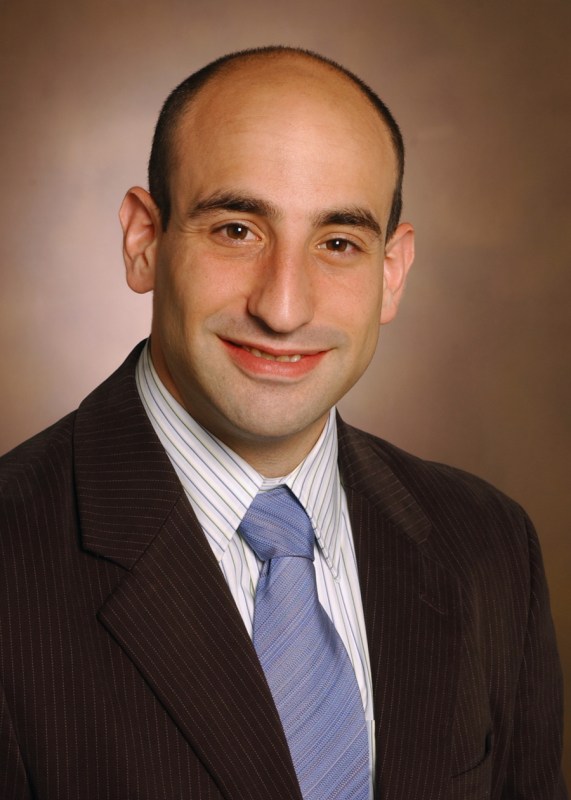
Brad Malin, Ph.D.
In a typical GWAS, computer algorithms are set to compare genetic profiles of subjects in clinically contrasting groups, those with and those without a given disease or sensitivity to a given drug. Patterns in the data may point to genes implicated in disease or to new genetic tests for predicting drug responses.
With the spread of electronic medical record-keeping, GWA studies are capturing extensive coded personal health information, making these data sets potentially useful for answering a range of hypotheses. Sharing and recycling of GWAS data can help speed the pace of biomedical discovery.
And in any given GWAS, there's also the more immediate issue of validation hinging on other teams taking a fresh look at the data.
Brad Malin, Ph.D., assistant professor of Biomedical Informatics, has previously asked whether there may be lingering privacy risks associated with conventionally anonymized clinical profiles — data that's already been carefully stripped of names, dates, zip codes, etc.
Malin poses a resourceful attacker who has already obtained your diagnosis codes, knows that you're represented in a given GWAS and has gained access to the study database. Malin has previously shown that such an attacker is very apt to be able to use the diagnosis codes to pick you out of the database and thus extract your linked genetic profile.
“We haven't made any claims in terms of who would mount such an attack; it was more an illustration that it's possible,” Malin said.
A new study by Malin and research fellows Grigorios Loukides, Ph.D., (the paper's first author) and Aris Gkoulalas-Divanis, Ph.D., appearing in the Proceedings of the National Academy of Sciences, introduces a computer algorithm that renders GWAS subjects less identifiable, while also preserving the usefulness of their clinical data for further research.
Diagnosis codes form a hierarchy, with multiple codes under asthma, diabetes and so on. Using data from an actual Vanderbilt GWAS, the authors generalize any overly conspicuous diagnosis code combinations by selectively substituting less conspicuous sets of related codes. They thus hide individuals within groups. At the same time, their algorithm protects certain codes from over-generalization.
In the study, the algorithm performs code substitutions to a point where no individual's codes correspond to a group of fewer than five people. The authors show that, even in this generalized state, the data are successfully kept unaltered for purposes of verifying the results of the GWAS. And what's more, they show that the data retain usefulness for studying a range of other diseases.
“The first thing that people want to be able to do is verify whether the data used in the published GWAS study really substantiates the claims within that study. We took it a step further and asked whether we could use the data to support other types of correlations. And we found that, instead of just validation, the data can be used for knowledge discovery.”
The method isn't limited to use with hierarchical coding structures. “The approach that we use is generalizable to medications, procedural codes, test results, whatever,” Malin said.
On a related note, a new study in the American Journal of Human Genetics gauges the usefulness Vanderbilt's repository of linked DNA samples and anonymized medical records, dubbed BioVU, for discovery of genotype-phenotype associations.
Marylyn Ritchie, Ph.D., associate professor of Molecular Physiology and Biophysics, Joshua Denny, M.D., M.S., assistant professor of Biomedical Informatics, and colleagues genotyped nearly 10,000 BioVU samples for gene variants associated with five separate disease groups.
When they processed the linked anonymized medical records, they found predicted levels of documented disease in disease groups and in control groups.