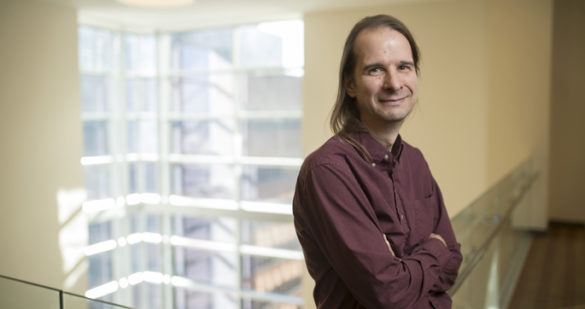
In a study in Circulation: Cardiovascular Genetics, Vanderbilt University’s Jonathan Mosley, M.D., Ph.D., and colleagues use genetic correlation to hitch together two unrelated sets of data, one from a longstanding epidemiological cohort and the other from electronic health records.
For studying disease risks, there are major limitations inherent in each of these types of data. This study overcomes them.
One limitation of prospective epidemiology is that “having enrolled your subjects and carefully gathered baseline data, you then have to wait 20 or 30 years to see who thrives and who gets sick,” said Mosley, research instructor in Medicine.
Conversely, the trouble with electronic health records is that, “While they may contain large numbers of clinically significant outcomes, such as heart attacks, they are frequently spotty in terms of baseline data essential for epidemiology, and they don’t contain data for novel or unproven risk factors and biomarkers.”
Some medical centers and some epidemiological studies these days have accompanying biorepositories and genotype data. Mosley’s study demonstrates how the availability of these data presents opportunities to answer epidemiological questions in weeks rather than decades.
This shortcut is provided by genetic correlation. If researchers genotype a large enough sample of the population, they can estimate the degree of overall genetic variability from one pair of individuals to the next, while noting the variability of any trait they care to measure.
“These two types of data let you estimate the effect of genes on the variability of traits. They won’t tell you which genes affect the traits you’ve measured, but you’ll be able to estimate the magnitude of genetic effects.”
These are all the data Mosley needs to calculate genetic correlations between traits. In this study, for example, he finds a 44 percent genetic correlation between total-to-HDL cholesterol ratio and ischemic heart disease, meaning there’s a 44 percent overlap in his sample between two unknown sets of causative genetic variants, one set partly explaining who gets high total-to-HDL cholesterol and the other set partly explaining who gets ischemic heart disease.
In two traits with a known genetic correlation, where one is captured in a simple clinical observation or test result and the other is captured in a chronic disease diagnosis, “There’s no need to wait around for decades to learn what association may or may not obtain between the two. With genetic correlation you can have an immediate view into a chronic disease risk,” Mosley said.
Epidemiologists have been measuring genetic correlations between pairs of traits for decades, but this required using related individuals and measuring each trait in each individual.
“With newer genetic technologies, we can use unrelated individuals and we only need to measure one of the traits in each individual,” Mosley said.
This route is far superior for finding traits genetically correlated with diagnoses. Crucially, so long as there’s a common well of genotype data from which to calculate the correlations, there’s no reason the traits can’t be measured in completely unrelated groups.
According to Mosley, the new study is the largest, most systematic demonstration of its type to date. He calculates genetic correlations between baseline measurements taken decades ago in 13,000 enrollees in a heart disease epidemiological cohort, and ischemic heart disease and type 2 diabetes documented recently within electronic health records of 25,000 patients.
The baseline measurements were gathered from 1987 to 1989 by the Atherosclerosis Risk in Communities cohort study (ARIC), and the electronic health record data come from the eMERGE Network and the Vanderbilt Electronic Systems for Pharmacogenomic Assessment cohort.
Fourteen of the baseline measures from the ARIC cohort bear genetic correlation with type 2 diabetes in the electronic health record group. For each of these baseline measures, association with type 2 diabetes in ARIC was measured, yielding 14 hazard ratios. Mosley finds that the paired hazard ratios and genetic correlations climb together, the greater the one, the greater the other. This confirms that, next time, he can calculate disease risks based on genetic correlation alone, saving 25 years of longitudinal research.
Ischemic heart disease (IHD) is a less well defined disease than type 2 diabetes, and the genetic correlation between IHD in ARIC and in the EHR group is accordingly weaker than is the case with diabetes. Nevertheless, eight of the ARIC baseline measures bear genetic correlation with IHD in the EHR group.
Here again, paired hazard ratios and genetic correlations climb together, but the correlations are weaker than with diabetes. Mosley widens the net, looking for genetic correlations between the baseline measures and other traits in the EHR group known to influence IHD. Compared to the ARIC cohort, IHD in the EHR group is more associated with triglycerides, blood pressure and HDL cholesterol, “Which suggests to me a phenotype of metabolic syndrome. This syndrome appears to be a stronger driver of IHD in the EHR population than was seen in ARIC.”
Many of the EHR group’s IHD cases and controls were seen at Vanderbilt. “This really allows us to say, ‘What if a study like ARIC had been done on our population? What unmet needs or what unmet risk are we not treating?’ This allows us to create a risk profile localized to our institution,” Mosley said.
Mosley looks forward to collaborating with other epidemiological studies, including upcoming research involving the well-known Framingham Heart Study.
“There are lots of groups doing large proteomic studies, large metabolomic studies, using brand new methods of measuring new proteins, new biomarkers, new everything, and now they have to wait 25 years to see if those biomarkers predict anything.
“We might be able to give you your outcome right now.”
Other contributors to the study from Vanderbilt include Sara Van Driest, M.D., Ph.D., Quinn Wells, M.D., PharmD, M.Sc., Christian Shaffer, Todd Edwards, Ph.D., Lisa Bastarache, M.S., Josh Denny, M.D., M.S., and Dan Roden, M.D.
Mosley’s work was supported by a career development award from the American Heart Association and by the National Institutes of Health (grants GM115305 and LM010685).