by Paul Govern
Electronic health records (EHR), in the aggregate, are increasingly a resource for biomedical discovery, and automated searches for records that reflect a phenotype of interest, typically a disease, are a common starting point.
Unfortunately, these searches aren’t straightforward in the least. For a given disease of interest, it takes time and effort for experts to devise, test and refine an electronic selection algorithm. These elaborate preliminaries are seen as slowing discovery.
To dramatically pick up the pace, a team at Vanderbilt University Medical Center is offering researchers everywhere, free of charge, a new tool they’ve devised called PheMap. In testing, PheMap’s accuracy proves comparable to or better than that of bespoke algorithms. The team introduced PheMap in a paper appearing in the recent issue of the Journal of the American Medical Informatics Association.
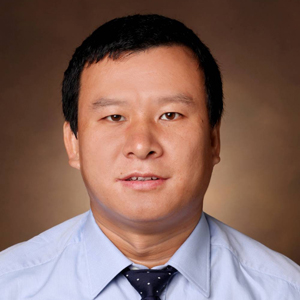
“PheMap provides a much needed high-throughput solution that can work right out of the box with any institution’s EHR, cutting the EHR phenotyping process down from months to less than half a minute,” said Wei-Qi Wei, MD, PhD, assistant professor of Biomedical Informatics, who led the work with Neil Zheng, an associate application developer in Wei’s lab. “We were particularly pleased with how well this all-purpose tool tested against hand-made algorithms.”
In previous work, a VUMC team led by Wei and Joshua Denny, MD, MS, had mapped EHR diagnosis-based billing codes to EHR disease phenotypes. That high-throughput system, called phenotype codes, or phecodes, has been used to scan for associations between common genetic variants and the full range disease phenotypes reflected in the EHR. PheMap leverages the phecode system and other clinical terminologies to achieve greater accuracy.
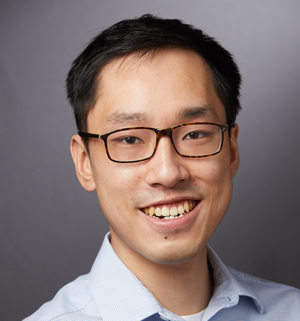
The team started with wholesale extraction of information from five of the world’s top consumer health web sites — MedlinePlus, MedicineNet, WikiDoc, Wikipedia, and Mayo Clinic Patient Care and Health Information — a massive collection overflowing with disease descriptions involving diagnoses, symptoms, treatments, lab results, medications, etc. Through a sequence of steps involving standard medical terminologies and natural language processing, they refined this giant corpus into a knowledge base of medical concepts mapped, in weighted fashion (based on frequency of association), via the preexisting phecode system, to a wide range of standard medical terminologies used throughout the EHR — diagnosis codes, procedural codes, symptoms, lab results, drug names and more.
With some 1,400 phenotypes currently in its quiver, in one quick scan of an EHR data base, PheMap can tell researchers who has a disease of interest and who doesn’t, that is, it can provide each record’s probability of having a phenotype, and this can easily be transferred into case/control status for a study.
The team compared PheMap to three alternatives: another all-purpose selection strategy called XPRESS; the preexisting phecode system on its own; and custom-built, clinician-validated selection algorithms. Using as a reference a set of bespoke algorithms for Type 2 diabetes, dementia and hypothyroidism, the accuracy of PheMAP for case and control selection was greater than 97%. For selection of these diseases it outperformed both XPRESS and the phecode system on its own.
For the same three diseases, the team compared genome-wide association study (GWAS) results using PheMap to results using clinician-validated selection algorithms. PheMap replicated 43 of 51 previously reported disease-associated variants, often providing more emphatic results, that is, significantly lower P values.
To again compare PheMap to the phecode system on its own, the team conducted side-by-side phenome-wide association studies (PheWAS) — where you start with genetic variants of interest and scan records for associated phenotypes. PheMap performed as well or better than the phecode system.
Other authors on the study included QiPing Feng, PhD, Eric Kerchberger, MD, Juan Zhao, PhD, Todd Edwards, PhD, MS, Nancy Cox, PhD, Michael Stein, MBChB, Dan Roden, MDCM, and Joshua Denny, MD, MS. The study was supported in part by the National Institutes of Health (HL133786, GM120523).