One of the monoclonal antibodies in the therapeutic Evusheld has been computationally redesigned to restore its action against Omicron variants of the virus that causes COVID-19.
The computational redesign approach, combined with experimental validation, could be used to rapidly address viral variants that are no longer blocked by antibody treatments, a multi-institutional team of researchers reported May 8 in the journal Nature.
“SARS-CoV-2 (the virus that causes COVID-19) is a difficult microbial target because the sequence of the virus is changing so fast that we face the need to update the antibody therapeutics that served us so well at the beginning of the pandemic,” said James Crowe Jr., MD, the Ann Scott Carell Professor and director of the Vanderbilt Vaccine Center (VVC), which experimentally tested the redesigned antibody candidates.
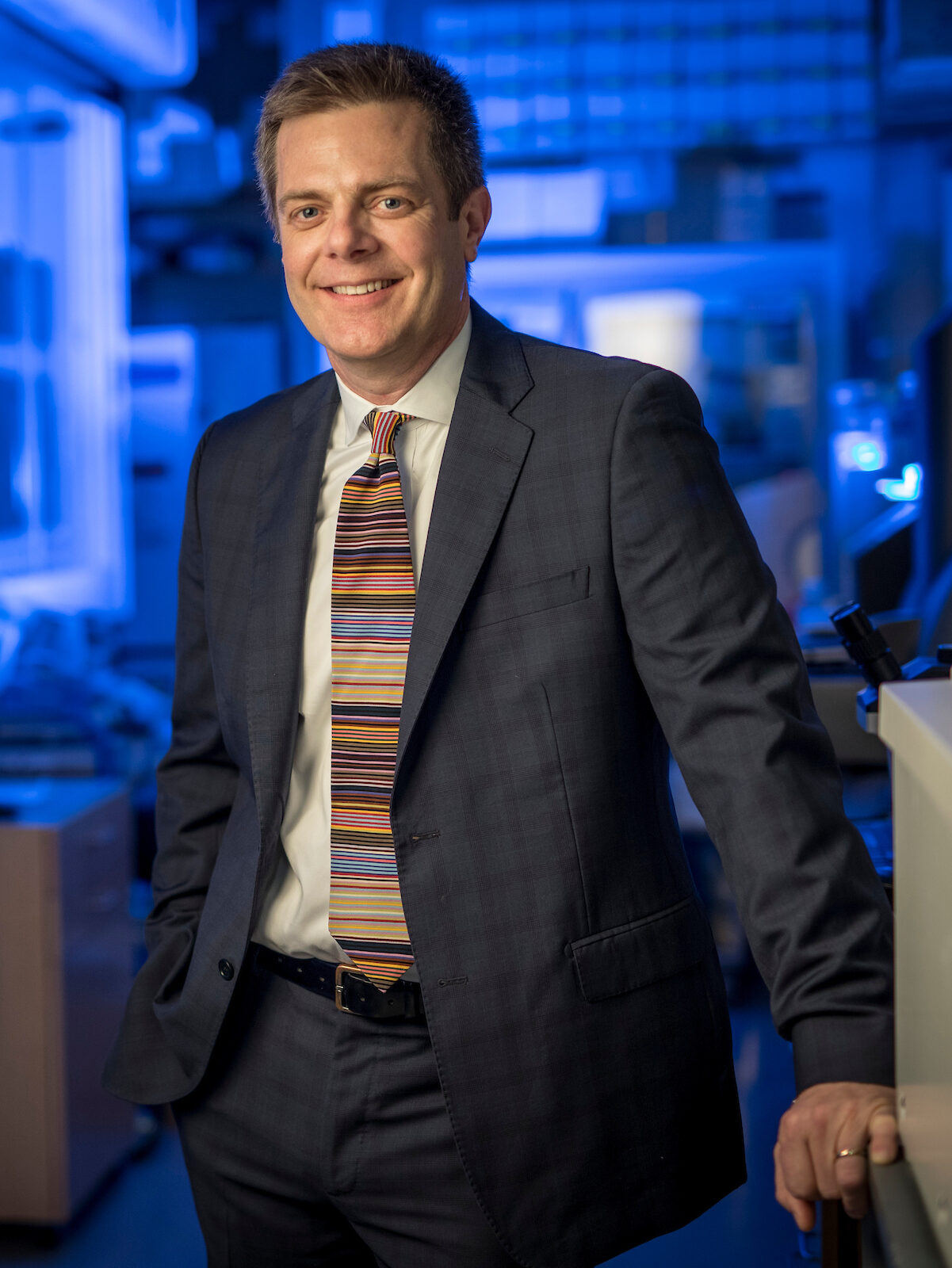
“The work performed here in this collaborative network rapidly accomplished a historic leveraging of supercomputing resources and expertise to update an important therapeutic model. Clearly, this is a new method for how we will keep antibody drugs up to date in the future against highly variable viruses.”
Investigators at Lawrence Livermore National Laboratory led the computational redesign effort.
Monoclonal antibodies seek out and bind to viral targets to destroy them, and the COVID-19 pandemic reinforced the promise of monoclonal antibody drugs as both prophylactic and therapeutic treatments for infectious diseases.
Crowe, Robert Carnahan, PhD, associate director of the VVC, and their colleagues have developed ultrafast methods to discover highly potent human monoclonal antibodies and validate their effectiveness. Evusheld is a combination of two monoclonal antibodies discovered at the VVC and optimized and developed by the global biopharmaceutical company AstraZeneca. It was the only antibody drug approved to treat high-risk immunocompromised patients before exposure to the virus that causes COVID-19.
The ability of the virus to mutate rapidly and the emergence of Omicron variants, however, allowed the virus to escape from many monoclonal antibody therapies, including Evusheld.
In the current study, the researchers used a computational redesign strategy to recover antibody efficacy against viral escape variants. They focused on one of the antibodies in Evusheld and sought to restore its ability to neutralize Omicron variants, while retaining its ability to neutralize a Delta variant. To do this, the team computationally introduced a small number of mutations into the part of the antibody that binds to SARS-CoV-2 and then assessed viral binding affinity of the redesigned antibody candidates.
The approach, called generative unconstrained intelligent drug engineering (GUIDE), combines high-performance computing, simulation and machine learning to co-optimize binding affinity to multiple targets, such as the binding domains from several different SARS-CoV-2 strains.
Steered by a machine learning model, the team simulated more than 125,000 antibody candidates, and then narrowed the set to 376 antibodies to experimentally determine binding to and neutralization of Delta and Omicron variants, thermostability, and in vivo prophylactic protection against the SARS-CoV-2 variants.
The best candidate, which had changes in only four amino acids of the antibody protein, had increased neutralization against Delta and Omicron variants and provided protection against infection in a mouse model.
“This collaborative work is a wonderful demonstration of how cutting-edge computational and wet lab approaches can come together to produce something neither could accomplish alone,” said Daniel Faissol, PhD, program leader for predictive design of biologics at Lawrence Livermore National Laboratory and the corresponding author of the Nature study. “Our response to the emergence of Omicron stood on the shoulders of giants by computationally optimizing an antibody discovered early in the pandemic with incredible speed by the VVC. There are countless ways in which VVC’s rapid discovery technologies and our in silico optimization can work together to produce exceptionally valuable antibodies, and I look forward to those continued collaborations.”
The researchers also used deep mutational scanning to demonstrate that the redesigned antibody candidate achieved improved potency without incurring other changes that would aid viral escape, and they used cryo-electron microscopy to detail the structural basis for restored antibody potency.
The authors note that their innovative computational approach could be rapidly deployed in response to emerging viral threats or evolutionary shifts in virus strains. The limited number of amino acid changes in the redesigned antibodies suggests that they may be eligible for accelerated regulatory approval if the parental antibody has already been approved for use in humans.
The GUIDE team is funded by the Department of Defense’s Joint Program Executive Office for Chemical, Biological, Radiological and Nuclear Defense. Major funding sources for the VVC include the DOD’s Defense Advanced Research Projects Agency and the National Institute of Allergy and Infectious Diseases, part of the National Institutes of Health.