With funding from the National Science Foundation, health information privacy experts at Vanderbilt University Medical Center, the University of Texas at Dallas and IBM have collaborated on a public case reporting framework keyed to the dynamics of pandemics. A report on this work from Thomas Brown, Bradley Malin, PhD, and colleagues appeared recently in the Journal of the American Medical Informatics Association.
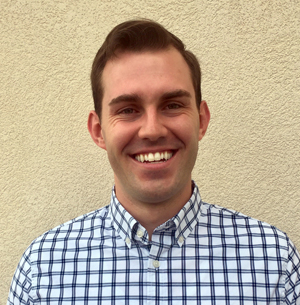
Hospitals and local public health authorities de-identify patient records as they share them with scientists or medical technology companies. Regulators look for de-identification methods that strip out names, geographical locations, birth dates, date of diagnosis, and so on.
“In data sharing, there’s an inherent tradeoff to be struck between patient privacy and data utility. But from the standpoint of managing a dangerous pandemic, routine suppression of dates and locations is apt to be a non-starter,” said Brown, a PhD student in Biomedical Informatics who works in Malin’s lab at VUMC, the Health Information Privacy Laboratory.
“More subtle, bespoke de-identification methods can allow smarter data sharing, but their form is apt to depend on the fixed size of a dataset assumed to already be in hand, making them unsuitable in a pandemic.”
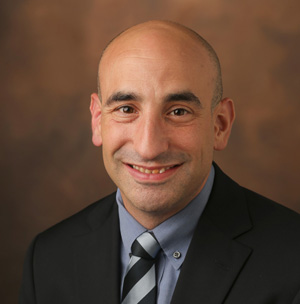
In the new report, a would-be privacy attacker is assumed to know a target is in the dataset in question, and knows not only the target’s demographic information, but also the time frame in which the target would have been evaluated for pandemic disease. Using this information, the attacker attempts to re-identify the target to learn sensitive information that may figure in pandemic case reporting, such as chronic disease diagnoses.
The framework set out in the report starts with combining a proposed public case reporting policy of given data granularity with summary demographic data from the U.S. Census Bureau. For demonstration purposes the report uses county-level data — the framework could also apply to states, zip codes, etc. Based on infection rates, pretend diagnoses are randomly injected into demographic data, producing a time series of synthetic pandemic patient datasets for a given county.
Re-identification risk going forward in a given county is estimated in terms of the proportion of synthesized pandemic patients who, by dint of sharing a unique set of demographic characteristics, are cast into anonymized groups smaller than size k, with k set for demonstration purposes to 11 people (in line with privacy standards advocated by public health authorities).
Optimal case reporting policies can be found by instantly applying multiple policies (and case number assumptions) and comparing the results.
The more people become infected the more granular case reporting can become without compromising privacy standards.
Using weekly county-level COVID-19 case number forecasts published by the Centers for Disease Control and Prevention, the study compares 96 alternative daily and weekly data sharing policies over a 15-month period for all U.S. counties with census tract data.
“We’ve laid out a dynamic framework that’s sensitive to case number forecasts and that allows policies to be redefined over time to prioritize public reporting of information such as diagnosis dates,” said Malin, Accenture Professor of Biomedical Informatics, Biostatistics, and Computer Science. “Our results show that, compared to the current state of the art methods that rely on retrospective data for anonymization, a dynamic and forward-thinking framework such as ours can enable timely public reporting while maintaining patient privacy.”
On the study also from VUMC were Chao Yan, PhD, Weiyi Xia, PhD, Zhijun Yin, PhD, and Zhiyu Wan, PhD. They were joined by Murat Kantarcioglu, PhD, of the University of Texas at Dallas, and Aris Gkoulalas-Divanis, PhD, with IBM in Cambridge, Massachusetts. The study was supported in part by the National Institutes of Health (LM007450).