Vanderbilt Genetics Institute investigators have added a new method to the computational genetics toolbox. Their approach, described in the journal Nature Genetics, integrates vast genomics datasets to predict gene expression and facilitate discovery of genetic mechanisms underlying human diseases.
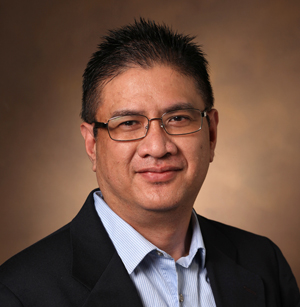
Since the introduction of genome-wide association studies (GWAS), investigators around the world have identified thousands of genetic variants associated with a range of complex diseases, such as type 2 diabetes, Alzheimer’s disease and coronary artery disease.
“The issue is that most of the identified variants are in non-coding regions of the genome (they are not located in genes that code for proteins), and this has made it enormously challenging to characterize the biological mechanisms responsible for disease,” said Eric Gamazon, PhD, assistant professor of Medicine. “We developed a computational framework for post-GWAS analysis, which we believe will open up new possibilities for research into these underlying mechanisms and causal gene-level relationships.”
The new method expands PrediXcan, a tool that Gamazon and his colleagues previously developed to correlate genetically regulated gene expression with phenotypes, for example the traits and diseases included in electronic health records. Whereas PrediXcan uses gene expression from a single tissue at one time, however, the new method integrates data from multiple tissues.
The improvement was made possible with data from the international Genotype-Tissue Expression (GTEx) Consortium, which has built an atlas across tissues. The latest GTEx data release was recently published in the journal Science.
“We have found through our involvement in GTEx that there is quite a bit of shared regulation of gene expression across tissues,” Gamazon said. “We leveraged this enhanced understanding of the genetic architecture of gene expression to improve our computational approach.”
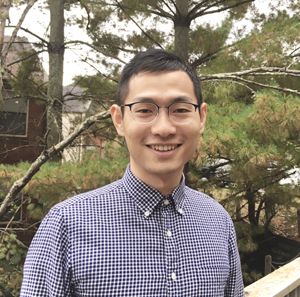
The investigators showed that the new method results in a “substantial improvement in the prediction of gene expression relative to PrediXcan,” Gamazon said.
To demonstrate how the tool can be used, the team applied it to low-density lipoprotein (LDL) cholesterol levels in a GWAS dataset from the UK Biobank. They confirmed known genetic associations and identified and replicated novel associations, expanding the list of genes associated with LDL cholesterol.
“Large datasets like the UK Biobank, BioVU and All of Us are growing bigger and bigger,” said Dan Zhou, PhD, research fellow in Genetic Medicine and first author of the Nature Genetics paper. “The methodology we developed maximizes our power to use these large-scale biobanks to detect associations with complex traits and diseases — to find more causal genes — and also to rule out false positive signals.”
The method will allow investigators to perform “in silico” randomized controlled trials with biobank data — to probe how a “treatment,” in this case gene expression, affects disease.
“We’re so excited about the new directions this approach will enable in human genetics studies. It will provide better resolution of causal effects of genes on complex diseases and facilitate prioritization of genes for functional validation studies,” Gamazon said.
Investigators involved in the research also included Yi Jiang, Xue Zhong, PhD, and Nancy Cox, PhD, from the Vanderbilt Genetics Institute and Chunyu Liu, PhD, at SUNY Upstate Medical University, Syracuse. Gamazon is a Life Member of Clare Hall, a graduate college devoted to advanced studies, research and scholarship at the University of Cambridge. This research was supported by the National Human Genome Research Institute, part of the National Institutes of Health (grants R35HG010718, R01HG011138).