Critical care patients are prone to shift intermittently into delirium or coma without warning. A team at Vanderbilt University Medical Center used machine learning to predict the likelihood of next-day brain function status changes in these patients.
The team’s report appears in the Journal of the American Medical Informatics Association.
“Time spent in the ICU with delirium or coma is associated with increased mortality and adverse long-term outcomes such as dementia. Reliable next-day forecasting of these patient transitions into and out of acute brain dysfunction could go a long way toward improving care and ICU resource allocation,” said the principal investigator of the study, You Chen, PhD, assistant professor of Biomedical Informatics.
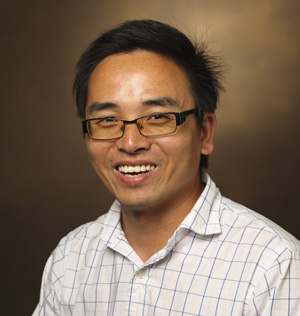
To build, test and validate their predictive model, the team used records from 1,026 adult ICU patients seen at five health care systems. Patients had been assessed for brain function status twice each day, with 71% experiencing delirium and 60% experiencing a coma during their ICU stay. Various machine learning strategies were tried and a light gradient boosting machine, a learning technique that’s as interpretable as linear regression, edged out competitors.
For a given binary medical outcome, the performance of a predictive model can be gauged by how likely it is to assign a higher risk score to a randomly selected patient who proves positive for the outcome than to a randomly selected patient who proves negative — a measure known as the area under the receiver-operating characteristic curve, or AUROC. With the outcome of interest being next-day delirium or coma, the team’s machine learning algorithm demonstrated an AUROC of 82.4%.
“As predictive models of patient outcomes go, an AUROC of 82% would generally be considered excellent — great enough to allow clinical teams to set cut-points that strike workable tradeoffs between true and false alarms,” Chen said. “And 82% turns out to be far superior to the next-best model we were able to find in the literature for this next-day outcome, which had an AUROC just below 70%.”
The team next devised a compact version of the algorithm, based on a handful of the most influential factors in their full model. With an AUROC of 81.9%, this second algorithm, virtually as accurate as the full version, is comparatively easy to adopt in clinical practice.
While the team’s full algorithm was trained on 369 patient variables, the compact model translates as 11 questions that clinicians can readily answer.
“Using artificial intelligence, we’ve hit upon a compact predictive model that’s quite practical to employ in critical care, and, based on our study sample, looks likely to be applicable to the general run of intensive care units,” Chen said.
Other Vanderbilt investigators on the study include lead author Chao Yan, a computer science PhD candidate, Cheng Gao, PhD, Ziqi Zhang, Wencong Chen, PhD, Bradley Malin, PhD, E. Wesley Ely, MD, and Mayur Patel, MD, MPH.
The study was supported by the National Institutes of Health (GM120484, HL111111).