Researchers at Vanderbilt University Medical Center and the Tennessee Department of Health (TDOH) have developed 30-day predictive models for fatal and non-fatal opioid-related overdose among patients receiving opioid prescriptions in the state.
The team applied machine learning techniques to statewide data sources that included details on 2,574 fatal and 8,455 non-fatal opioid-related overdoses occurring within 30 days of an opioid prescription. In all, the data involved just over 3 million patients and more than 71 million prescriptions for controlled substances.
The team’s report appeared Oct. 19 in the Journal of the American Medical Informatics Association.
According to TDOH, there were 3,032 overdose deaths in Tennessee in 2020, a 45% increase from 2019. Opioids, both illicit and prescribed, were involved in 79% of the state’s overdose deaths in 2020, and 19% of Tennesseans who died of a drug overdose in 2020 had an opioid prescription in the 60 days before death.
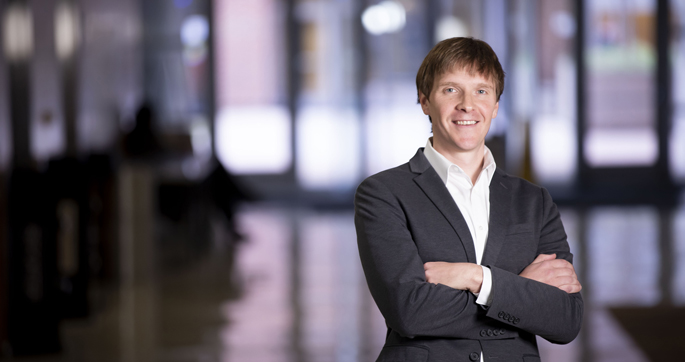
To assess and engage the opioid overdose crisis, public health authorities in Tennessee have relied solely on current and retrospective descriptive data, without prognostication.
According to the report’s senior author, Colin Walsh, MD, MA, associate professor of Biomedical Informatics, Medicine, and Psychiatry and Behavioral Sciences, TDOH will continue to study the predictive models with an eye to their potential deployment in the public heath response to the ongoing crisis.
The new models are not designed to guide interventions at the patient level. By predicting risk at the prescription level, the models are instead designed to allow aggregation of risk to practices and health care organizations, pharmacies, localities and counties.
“With these predictive models, Tennessee might bolster overdose prevention through better understanding of systems, communities and regions at highest risk before overdoses have occurred,” Walsh said. “If this were to prove useful in Tennessee, the tools and methods we’ve used could potentially be adapted by other states to support more targeted prevention.”
According to Ben Tyndall, PhD, a director with TDOH’s Office of Informatics and Analytics and a co-author of the report, TDOH is working in the longer term toward more granular risk quantification to improve prevention targeting for underserved populations.
“The Tennessee Department of Health is continually seeking innovative ways to leverage the myriad data sources available to the state to tackle the epidemic of drug overdose,” Tyndall said. “The collaboration with Dr. Walsh’s team has been a fantastic opportunity to join our public health experience and knowledge of the data with the methodological expertise at VUMC, and we are excited about the possibilities that will emerge as we continue this work.”
According to the report, three other states, Maine, Maryland and Oregon, have used state records to predict overdose risk.
As prediction of patient outcomes go, on technical measures both models perform in a range that is generally considered excellent. The data used to train and test the models came from Tennessee’s Controlled Substance Monitoring Database, the state’s Hospital Discharge Data System, death records maintained by the state and publicly available socioeconomic indicators related to health and health care utilization and access.
“This study is a fantastic example of how collaboration between academic medicine and public health can allow researchers and public health practitioners to bring the best tools to the fight against opioid overdose. By using methods typically applied in medical care for patients, we can think of communities or other entities as the focal points where resources can be directed,” said report co-author Melissa McPheeters, PhD, MPH, adjunct professor of Biomedical Informatics and Health Policy and senior scientist at RTI International (also known as Research Triangle Institute), headquartered in Research Triangle Park, North Carolina.
Joining Walsh in leading the project was first author Michael Ripperger, an application developer in Walsh’s lab; others from VUMC on the study include Sarah Lotspeich, PhD, Drew Wilimitis, Carrie Fry, PhD, Matthew Lenert, Katelyn Robinson and Qingxia Chen, PhD. Others from TDOH on the study include Allison Roberts, PhD, Sanura Latham, and Charlotte Cherry, MS, MPH.