Acute kidney injury, or AKI, is a common complication of cardiac catheterization, posing higher costs, longer hospital stays and increased short-term and long-term mortality risk.
In a large, randomized trial conducted over a period of 18 months at 19 medical centers of the Veterans Health Administration, a quality improvement implementation trial found a 46% reduction in the odds of AKI after cardiac catheterization.
Led by researchers at Vanderbilt University Medical Center, the Dartmouth Geisel School of Medicine, in Hanover, New Hampshire, and the Larner College of Medicine at the University of Vermont, in Burlington, the trial was reported Jan. 13 in the Clinical Journal of the American Society of Nephrology.
Each year in the United States, interventional cardiologists perform more than a million cardiac catheterizations for diagnostic or therapeutic purposes, threading a thin, flexible tube through the circulatory system and into a chamber or vessel of the heart. As many as 14% of patients experience AKI from the procedure, due in various measure to exposure to the contrast material used in fluoroscopy (this technology allows clinicians to see the catheter’s path as it enters the heart), ischemic injury arising during the procedure, and kidney disease and other underlying patient problems.
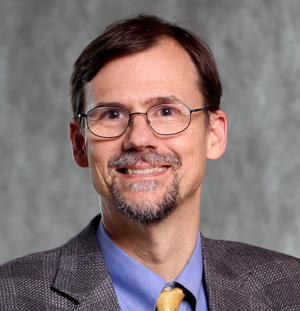
In the trial, four improvement strategies were compared across different medical centers: a modicum of technical coaching alone, a more thoroughgoing virtual learning program alone, and those two interventions separately wedded with access to an electronic dashboard providing monthly observed-to-expected AKI rates.
In all, the trial involved 4,517 cardiac catheterization patients, 510 of whom experienced AKI following their procedure.
“While some interventions, such as hydration and limiting contrast, are well known to reduce AKI risk, it can be challenging for all facilities to implement identical protocols,” said a leader of the study, internal medicine specialist and observational data scientist Michael Matheny, MD, MS, MPH, professor of Biomedical Informatics at VUMC. “This implementation trial facilitated each site team to make their own decisions based on quality improvement coaching and availability of case series, outcomes, and awareness of local case mix. It was really exciting to see different clinical foci and different process changes across sites show improvements in overall AKI event rates through the use of the various interventions.”
Joining Matheny to lead the study were Jeremiah Brown PhD, professor of Epidemiology at Dartmouth, and Richard Solomon, MD, professor of Medicine at Vermont.
The AKI rate was 13% among medical centers using either technical coaching alone or virtual learning alone.
The rate was 11% when the outcomes dashboard was wedded to light technical coaching, and 8% when the dashboard was wedded with virtual learning.
The change from 13% to 8% amounts to a 38% drop in AKI, and in data adjusted for patient severity, this came to an estimated 46% reduction in AKI risk.
The electronic dashboard was powered by an AKI prediction algorithm developed by Matheny and his team at VUMC, using machine learning and electronic health record data from all 76 cardiac catheterization centers in the VA.
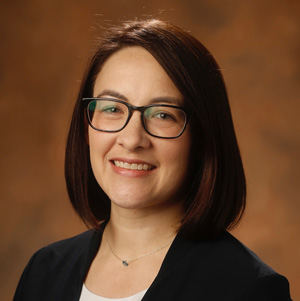
“The dashboard included data visualizations and gave clinicians the ability to drill into their patient cases and look at their individual outcomes,” Matheny said. “We were also able to deliver summary comparative performance metrics to each participating center. I began to develop some of the informatics methods for this trial as a VA career development awardee almost 15 years ago. It is very exciting to work with a large multi-site collaborative team to leverage that work to directly impact patient lives and outcomes.”
Matheny singled out the contributions of Sharon Davis, PhD, MS, research assistant professor of Biomedical Informatics at VUMC, who led a team to maintain the performance of algorithms used in the trail.
“From a data science perspective, one of the most exciting parts of this trial was a set of machine learning surveillance and updating algorithms developed by Dr. Davis. This trial started right before the COVID pandemic, which included unprecedented shifts in data, cath lab closures and re-openings, and changes in case mix and process. Her methods saved the day and allowed the risk adjustment models to maintain performance and be usable for sites throughout the course of the pandemic.”
Others on the study from VUMC include Dax Westerman, MS, Chad Dorn, PSM, and Freneka Minter, PhD, MS. The study was supported in part by the National Institutes of Health (HL157130).