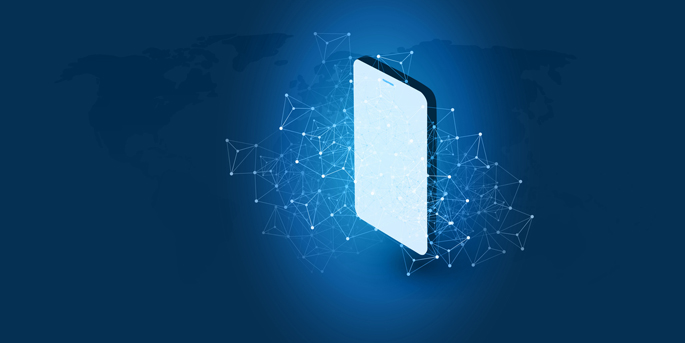
A recent study finds promise in the artificial intelligence program ChatGPT for speeding review and improvement of computer system alerts used to support day-to-day clinical decision-making.
In a blinded test at Vanderbilt University Medical Center, a panel of four physicians and one pharmacist reviewed suggestions from ChatGPT 3.5 mixed with suggestions from teams of clinical specialists, the test involving seven alerts from among the myriad logic-based alerts in use at VUMC.
The judges, rating suggestions on a scale of one to five, gave an average rating of 3.6 to suggestions from clinical specialists, 3.3 to suggestions from ChatGPT. They rated 65 suggestions in all, 36 composed by ChatGPT, 29 by clinical specialists. Of the test’s 20 top-rated suggestions, nine were from ChatGPT.
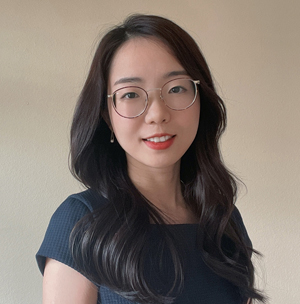
The test, led by Siru Liu, PhD, a postdoctoral research fellow in the Department of Biomedical Informatics, and Adam Wright, PhD, professor of Biomedical Informatics and Medicine and director of the Vanderbilt Clinical Informatics Center, was reported in the Journal of the American Medical Informatics Association.
“Across health care, most of these well-intentioned automated alerts are overridden by busy users. The alerts are seen as serving an essential purpose, but the general need to improve them is clear to everyone,” Liu said. “It’s apparent to me that AI could help speed this continuing project. ChatGPT appears already highly useful, and with specialized training it could no doubt be made yet more formidable for this vital purpose.”
A large language model optimized for dialogue, trained on web pages and books, ChatGPT 3.5 is an artificial neural network with some 175 billion parameters, or weighted connections analogous to
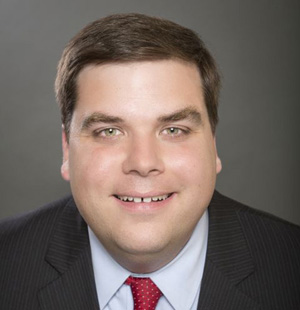
synapses (of which the human brain has more than 100 trillion). The chatbot was released last November by OpenAI, based in San Francisco. This March saw the introduction of the company’s yet more capable and headline grabbing ChatGPT 4.
Coming from various companies, Wikipedia lists 15 large language models having 100 billion or more parameters.
In the test, all members of the blinded panel had formal training in informatics and experience optimizing clinical decision support tools. The alerts involved contraindications for various drugs and clinical tests, post-operative patient risks, and patient documentation needed for prescribing and for patient management more generally. All suggestions received separate scores for usefulness, relevance, understanding, bias, redundancy, need for editing, implications for improved workflow, and inversion (that is, whether any suggestions appeared to run counter to the sense of the prompt).
Questions loom concerning how quickly large language models like ChatGPT might be coaxed into guiding clinical documentation or even decision-making on a more open-ended, patient-by-patient, problem-by-problem basis.
“We can look forward to an AI-powered transformation of health care occurring over time,” Wright said, “and in terms of raw technological capacity, with the success of large language models like ChatGPT perhaps the timeframe appears to be shrinking. But getting there will require building processes to ensure not only safety and efficient workflow, but fairness across diverse patient populations. That’s apt to be a quite thorny and drawn-out task.”
Others on the study from VUMC include Aileen Wright, MD, MS, Barron Patterson, MD, Jonathan Wanderer, MD, MPhil, Scott Nelson, PharmD, MS, and Allison McCoy, PhD. They were joined by researchers from the University of Texas Southwestern Medical Center in Dallas and the University of Texas Health Science Center in Houston. The study was supported by the National Institutes of Health (LM014097).