In a new study, researchers at Vanderbilt University Medical Center have demonstrated the potential for large language models like ChatGPT to help generate electronic health record (EHR) phenotyping algorithms, a critical but time-consuming task in observational health research.
The findings, reported April 13 in the Journal of the American Medical Informatics Association, suggest these artificial intelligence tools could help accelerate discovery.
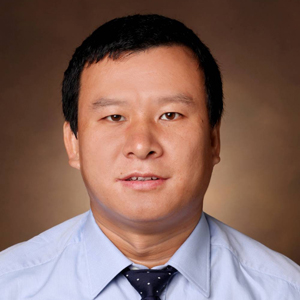
EHR phenotyping involves creating complex electronic algorithms to identify patients with specific characteristics by integrating clinical data like diagnoses, medications, procedures, and lab results. Traditionally, this requires extensive input from experts, including a thorough literature review and evidence synthesis, making it both time-consuming and resource intensive.
“Developing EHR phenotypes demands substantial informatics and clinical knowledge. It’s an intricate process that limits the pace of research,” said corresponding author Wei-Qi Wei, MD, PhD, associate professor of Biomedical Informatics. “We wanted to see if large language models could help generate preliminary algorithm drafts to make phenotyping more efficient.”
The team tested the ability of Open AI’s ChatGPT-4 and -3.5 models, as well as Claude 2, from Anthropic, and Bard (more recently renamed Gemini), from Google, to generate phenotyping algorithms for type 2 diabetes, dementia, and hypothyroidism. The algorithms were intended to search and analyze data from electronic health records and identify patients with these three conditions; for this pilot study, algorithms were generated using default versions of each of the four AI models (as available in October 2023).
Three phenotyping experts evaluated the algorithms, finding that ChatGPT-4 and -3.5 significantly outperformed Claude 2 and Bard in generating accurate, executable algorithms.
“GPT-4 and GPT-3.5 did well at finding appropriate diagnosis codes, lab tests and medications for these diseases,” said first author Chao Yan, PhD, a postdoctoral research fellow in Biomedical Informatics. “They even suggested additional criteria like symptoms that aren’t typically used in these algorithms but appear quite plausible.”
However, the generated algorithms still contained some errors, like incorrect code types, missing codes or imprecise lab thresholds. The models also sometimes applied overly broad or restrictive logic, resulting in algorithms that would identify too many or too few patients.
When the team tested their top-rated AI-generated algorithms on EHR data from more than 80,000 patients, they found mixed performance compared to gold-standard algorithms developed by experts. While some achieved high accuracy for case identification, others were far less precise.
According to the authors, the results highlight the potential of large language models as a tool to accelerate phenotyping by providing a useful starting point.
“These AI models show exciting capabilities, but they’re not yet ready to generate expert-level phenotyping algorithms, certainly not right out of the box,” Wei said. “We believe they can help jumpstart the process, allowing experts to focus more on fine-tuning rather than starting from scratch. It’s a promising human-computer collaboration.”
The researchers plan to further optimize prompts and explore emerging language models to advance the approach.
Others on the study included Henry Ong, PhD, Monika Grabowska, MS, Matthew Krantz, MD, Wu-Chen Su, MS, Alyson Dickson, MA, Josh Peterson, MD, QiPing Feng, PhD, Dan Roden, MD, C. Michael Stein, MD, V. Eric Kerchberger, MD, and Bradley Malin, PhD.
The study was supported by the National Institutes of Health (grants R01GM139891, R01AG069900, F30AG080885, T32GM007347, K01HL157755, U01HG011181).