Can algorithms peer into medical records and predict who will develop bipolar disorder? In a new study across three major U.S. health care systems, researchers developed and tested several predictive models, finding feasibility despite challenges.
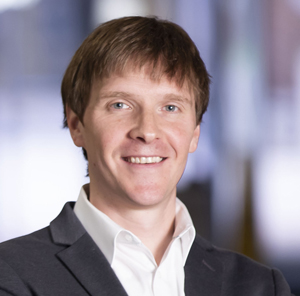
Bipolar disorder is a mental illness that causes severe shifts in mood, energy, activity levels and concentration. According to the National Comorbidity Survey Replication study, which uses a broad definition of bipolarism, an estimated 4.4% of U.S. adults experience bipolar spectrum disorders at some time in their lives. Posing risks for disability, premature mortality and suicide, bipolar disorder is underdiagnosed and thus often mismanaged.
“Those with bipolar disorder often suffer delays in accurate diagnosis; in some cases, they may not be correctly diagnosed for over a decade. Our study suggests predictive models applied to data in medical records might help timely diagnosis,” said lead author Colin Walsh, MD, MA, associate professor of Biomedical Informatics, Medicine and Psychiatry at Vanderbilt University Medical Center. “Our results point a way toward reducing diagnostic delay and might prompt earlier appropriate treatment for these patients.”
Reported in Translational Psychiatry, the study leveraged extensive electronic health record data on 3.5 million patients, including more than 12,000 diagnosed bipolar cases, across Vanderbilt, Geisinger Health System, based in Danville, Pennsylvania, and Mass General Brigham, based in Boston.
The investigators tested three machine learning approaches: random forests, gradient boosting machines and penalized regression. Each algorithm was trained at one site and then validated at the other two sites. An ensemble approach stacking all three algorithms was also assessed.
Optimal metrics were seen when algorithms were validated at their original training site, showing the difficulty of portability across health care systems. As Walsh noted, comparison of the algorithms “indicated that an ensemble approach provides the best performance overall but required local retraining.”
Across the three centers the top-performing models tested 82% to 87% accurate in terms of ranking patients according to risk, suggesting a high degree of clinical usefulness. For each model, the team examined positive predictive value, or PPV — the ratio of true positives to total (true and false) positives — with reference to various risk cutoffs. For patients in the top percentile of risk per the ensemble method, PPV across the three centers ranged from 4.5% to 6.8%.
The models relied on demographics, diagnostic codes and medications — EHR variables that readily fall into tables and thus lend themselves to computation. Walsh said they purposefully avoided free text or imaging data to ease eventual implementation.
“Our algorithms showed encouraging performance, but translating predictive models across health care systems remains an uphill battle,” he said. “We’re making our models open source to fuel wider validation. If they prove consistently useful in flagging bipolar risk, we’ll have an important tool to accelerate research and help guide screening and preventive care — but we aren’t there yet. Our next step is to test the potential of these algorithms to reduce diagnostic delay. Some individuals with bipolar disorder are initially diagnosed with unipolar depression and prescribed antidepressant that might inadvertently induce mania.”
The study was led by investigators from Vanderbilt, Geisinger and Mass General Brigham as part of the PsycheMERGE research network linking extensive EHR data to biobanks across leading academic medical centers. The paper’s senior author is Jordan Smoller, MD, of Harvard University and Mass General Brigham.
The VUMC site lead for PsycheMERGE is Lea Davis, PhD, associate professor of Medicine in the Division of Genetic Medicine. Others on the paper from VUMC include Davis, Michael Ripperger, Hyunjoon Lee, MS, and Drew Wilimitis. PsycheMERGE is supported by the National Institutes of Health (R01MH118233).