An artificial intelligence (AI) tool developed at Vanderbilt University Medical Center accurately identified pediatric patients at high risk for blood clots in a clinical trial. However, there was no difference in outcomes compared to a control group, the researchers reported Oct. 13 in JAMA Network Open.
One reason this may have occurred was that the recommendation to begin blood-thinning therapy in these patients were accepted by treating physicians less than 26% of the time. The treating physicians expressed concern that the therapy might cause a major bleed, although this complication was not observed during the study.
Although the outcome was surprising, the researchers said the clinical trial, called CLOT (Children’s Likelihood of Thrombosis), confirmed the safety and effectiveness of the tool in a healthcare setting, and provided valuable insights into how to incorporate it successfully into clinical practice.
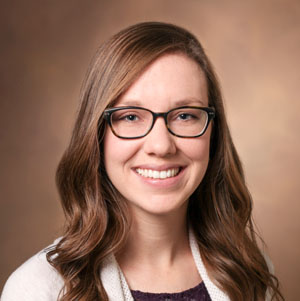
“There is going to be more and more AI in healthcare. Having a system established where we can assess these (models) will allow us to provide safer and more effective care to our patients,” said Shannon Walker, MD, assistant professor of Pathology, Microbiology and Immunology and Pediatrics, and the paper’s first author.
“This study demonstrates that a pragmatic patient-level, randomized, controlled trial is the most ethical and effective way to assess whether AI tools are safe and effective,” added co-author Daniel Byrne, MS, director of AI Research at the Advanced Vanderbilt Artificial Intelligence Laboratory (AVAIL) and the Department of Biostatistics.
Although rare, blood clots that develop in pediatric patients can lengthen their hospital stay and increase the risk of post-discharge complications and death.
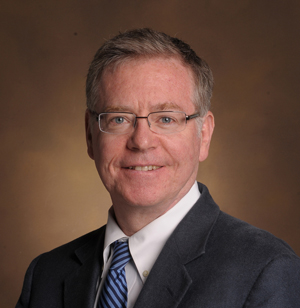
To identify those at high risk, Walker, Byrne, and their colleagues pored over the electronic medical records of more than 110,000 admissions to the Monroe Carell Jr. Children’s Hospital at Vanderbilt.
They identified 11 factors associated with blood-clot risk, including certain lab values and diagnoses, and whether the patient had undergone surgery or had cardiology or infectious disease consults.
Using that information, they developed a predictive model that, by scanning the medical record automatically, calculated a risk score daily for every pediatric hospital admission. “This allowed us to quickly review over 100 patients a day and focus on patients who had the highest likelihood of developing blood clots,” Walker said.
The trial, which ran for 15 months, from November 2020 through January 2022, included 17,000 hospitalizations. Patients were randomly divided into two groups. Risk scores for the study group were shared with their treatment teams; scores for those in the control group were not.
In the study group, scores for high-risk patients were accompanied by recommendations to initiate anti-thrombolytic therapy to prevent the development of blood clots. Patients in the control group, identified as high risk by their treating physicians without relying on the automatically generated risk score, also received blood thinners.
No bleeding complications were observed in any of the patients receiving blood thinners per the study recommendations.
At the conclusion of the trial, the researchers found no difference in the rate of blood clots between the study and control groups. An analysis of the medical records revealed the recommendation to begin blood thinners in high-risk patients in the study group was followed only 25.8% of the time.
“Without performing the trial,” Walker said, “it would have been impossible to identify potential reasons the intervention was unsuccessful.” It wasn’t a failure of the model, she added, but could have been due to reluctance to accept the recommendations.
The application of AI to clinical practice has been met with its share of pushback, Walker said. Skeptics have argued that implementation and evaluation of the algorithms, the “nuts and bolts” of the AI tools, is not feasible, too time-consuming, and will sap already limited clinical resources.
The CLOT trial showed that results can be obtained rapidly, without taxing the time or resources of the treatment team, by automatically randomizing and enrolling patients in a clinical trial using information that already exists in the electronic medical record.
It demonstrated the feasibility of assessing the value of predictive AI models in health care. But more work needs to be done.
Walker, Byrne, and their colleagues are planning another trial to understand better the reluctance of providers to accept the recommendation to begin blood-thinning therapy in patients at high risk for blood clots, and how to overcome it.
“Vanderbilt is performing rigorous science to ensure that these AI tools are safe and improve outcomes before we claim this. Other hospitals and AI vendors are skipping the science,” noted Byrne, author of a new book, Artificial Intelligence for Improved Patient Outcomes – Principles for Moving Forward with Rigorous Science.
“We need to make sure these models are performing as expected,” Walker added. “The infrastructure from this trial will allow for large study populations, to determine whether interventions that use artificial intelligence are safe and effective, and to help identify the patients who may benefit the most.”
Walker and Byrne’s co-authors were Benjamin French, PhD, Ryan Moore, MS, Henry J. Domenico, MS, Jonathan Wanderer, MD, MPhil, Amanda Mixon, MD, MS, MSPH, C. Buddy Creech, MD, MPH, and Allison Wheeler, MD, MSCI.
The CLOT study was the Advanced Vanderbilt Artificial Intelligence Laboratory’s first demonstration project. Partial support was provided by grant UL1TR002243 from the National Center for Advancing Translational Sciences, National Institutes of Health.